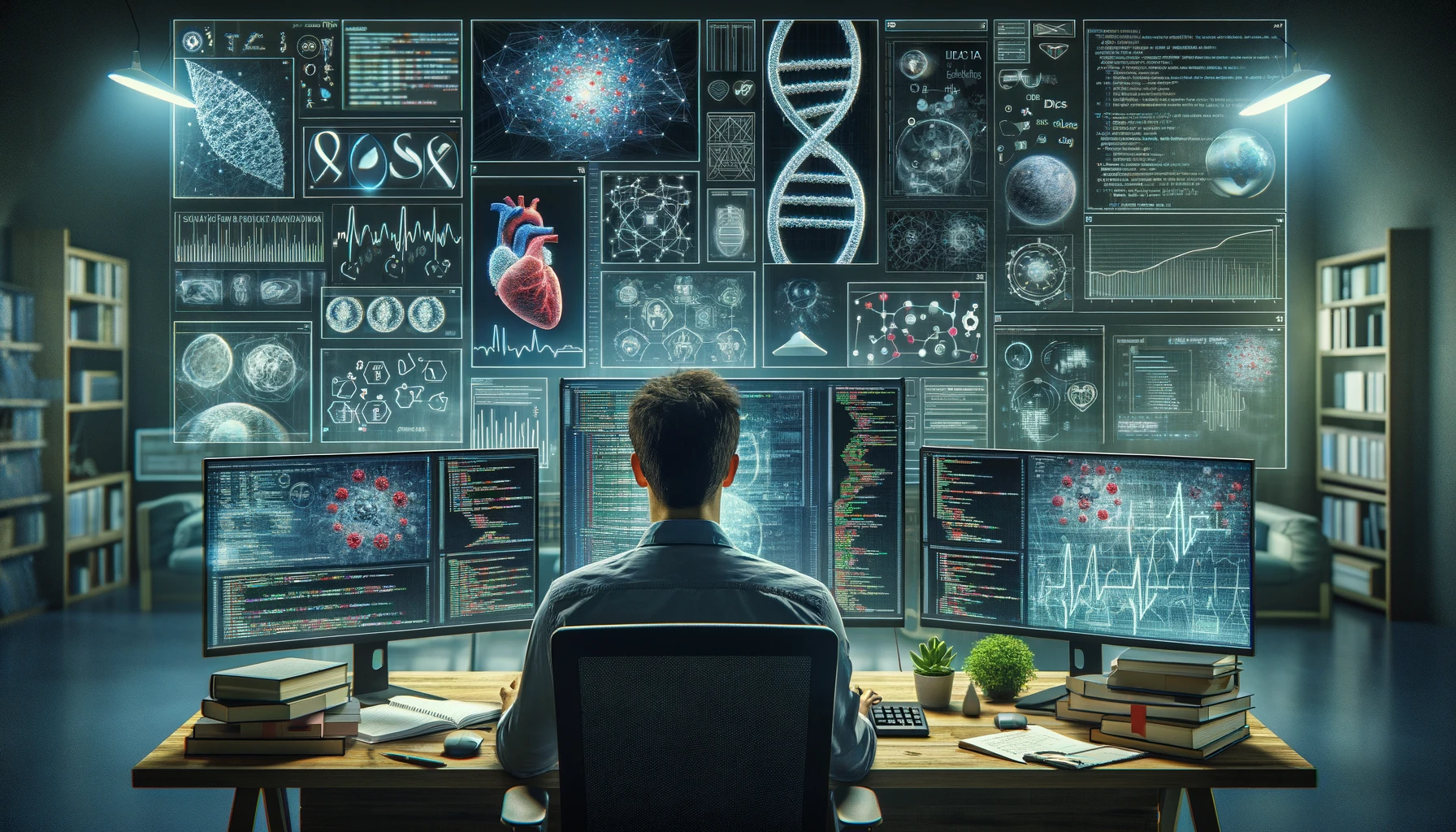
Identifying and accessing the right healthcare datasets for machine learning can transform how we approach diagnostics, treatment, and predictive analytics. Our guide dives into where and how to find datasets ranging from patient EHRs to genomic research, and how they’re driving innovation in healthcare analytics. Whether you’re developing models or looking for data to support your research, we deliver the insights you need without the fluff.
Key takeaways
- Healthcare datasets are vital for ML innovations, enabling the development of predictive analytics, personalized treatment plans, and enhanced diagnostic accuracy.
- Privacy-preserving methods are essential in handling sensitive healthcare data responsibly, using anonymization, de-identification, and synthetic data to maintain patient confidentiality.
- Access to healthcare datasets is facilitated through open data initiatives, repositories, collaborations with healthcare providers and pharma, with practical ML applications evident in predictive analytics and personalized medicine.
Essential healthcare datasets for machine learning innovation
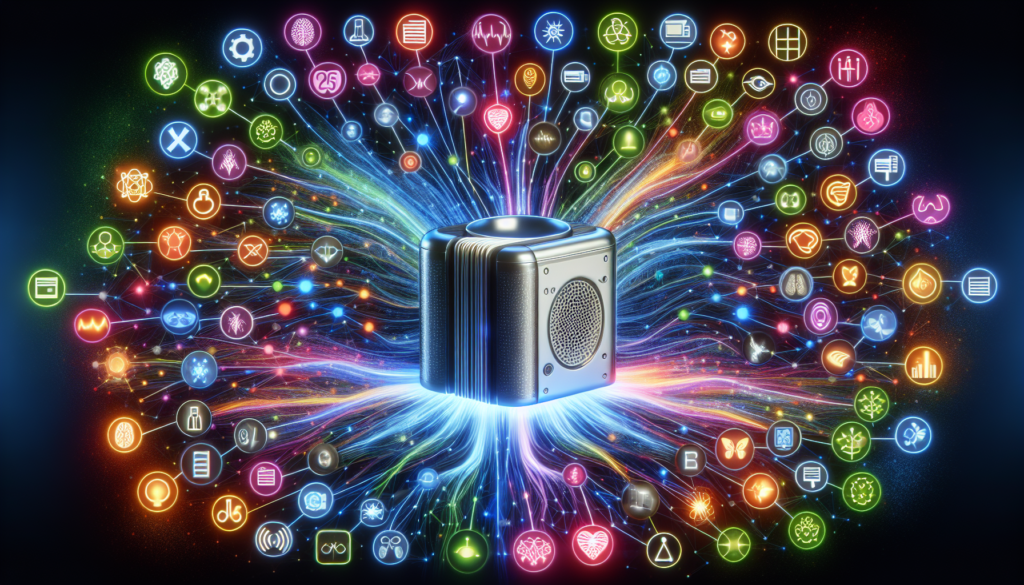
Machine learning and healthcare are two fields that have been intertwined for years, yet the depth of their connection is only just being discovered. At the intersection of these two fields lies a treasure trove of healthcare datasets, each with the potential to uncover significant advancements in the industry. Data scientists play a crucial role in analyzing these datasets and applying machine learning techniques to drive innovation.
From hospital care analytics to medical images, from large sets of hospital stays to quality of care information, the spectrum of healthcare data is vast. However, it’s not merely the volume of data that matters. The true value lies in the datasets’ capacity to bolster machine learning model development and refine algorithms. The validation of synthetic healthcare datasets, which includes qualitative analysis, hierarchical statistical testing, and evaluation of synthetic versus real data, ensures realism and security.
The goldmine of healthcare data is vast and varied, offering a plethora of opportunities to develop innovative machine learning models. The pivotal question is how to extract valuable insights from this data. We need to identify the necessary tools and their appropriate applications. We will examine three specific types of healthcare datasets: Electronic Health Records (EHRs), Imaging Data Repositories, and Genomic and Genetic Research Troves.
Electronic health records (EHRs) as a goldmine for ML
Electronic Health Records (EHRs) are essentially digital versions of patients’ paper charts. They contain a wealth of patient data, from vital signs to demographics, and are one of the most sought-after types of healthcare datasets. The largest publicly available collection of de-identified EHRs related to intensive care unit (ICU) patients is managed by the MIT Laboratory for Computational Physiology and is known as MIMIC-III.
However, the analysis of EHRs can be challenging as they often contain unstructured medical data. This is where Natural Language Processing (NLP) techniques come to the rescue, analyzing the unstructured data in EHRs and enhancing patient care and treatment outcomes. The automation of information extraction from EHRs, such as diagnoses, medications, and symptoms, presents opportunities for machine learning applications, although it also presents challenges like data privacy and the handling of unstructured data.
Imaging data repositories: from X-rays to MRI
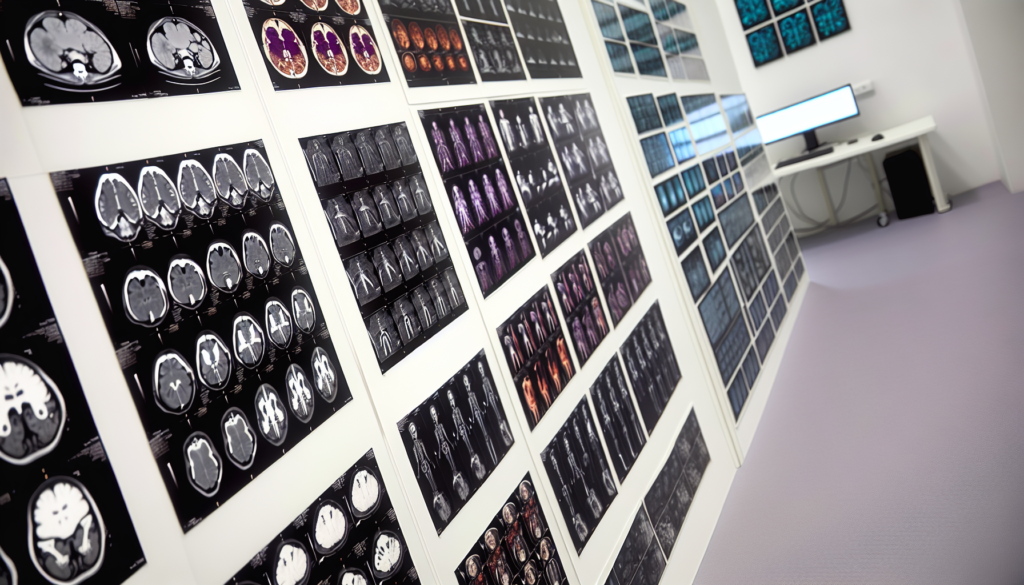
Diving deeper into the goldmine, we come across a different kind of treasure – imaging data. These datasets, including the Cancer Imaging Archive (TCIA), Alzheimer’s Disease Neuroimaging Initiative (ADNI), and OpenNeuro, provide essential data for machine learning models focused on medical imaging and disease detection. By utilizing the Alzheimer’s Disease Neuroimaging Initiative, researchers can enhance their machine learning models and improve early detection of Alzheimer’s disease.
The TCIA, for instance, is an extensive collection of de-identified radiology and histopathology images for various types of cancer. Meanwhile, the ADNI dataset offers MRI and PET images, along with genetics and biomarker information focused on Alzheimer’s research. These databases not only offer a wealth of data for machine learning models but also contribute to the scientific community’s understanding of diseases and their characteristics.
How do we apply these imaging datasets in a practical setting? One study, for instance, utilized imaging data from TCIA to study the accuracy of deep learning algorithms for diagnosing HPV in CT images of advanced oropharyngeal cancer. Another example is the NIH Database of Chest X-Rays, a large imaging collection with over 112,000 images. These practical applications highlight the value of imaging data in healthcare machine learning.
Genomic and genetic research troves
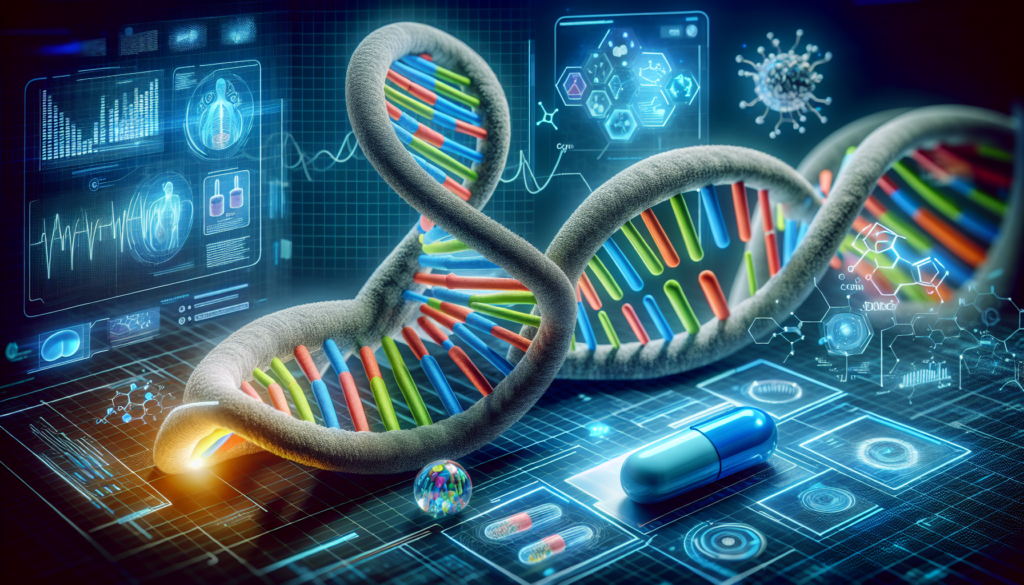
Venturing further into the healthcare data landscape, we discover the invaluable assets of genomic and genetic research collections. The 1000 Genomes Project, for instance, is an international collaboration that has created an extensive catalog of human genetic variation. By examining the DNA from 2500 individuals across 26 unique populations, the project has identified over 80 million variants.
The information contained within the 1000 Genomes Project dataset, such as single nucleotide polymorphisms (SNPs), structural variants, and haplotype information, is crucial for studying genetic differences. Furthermore, it has been used in precision medicine, such as in genotype-guided dosing of warfarin, showcasing the personalized medication plans enabled by AI.
Machine learning applied to pharmacogenomics leverages patients’ genetic makeup for tailoring drug treatments, enhancing drug efficacy, and reducing adverse side effects.
Leveraging public health data for ML predictive analytics
Although healthcare datasets have immense potential for machine learning development, their true potential is realized when they are put to practical use. How can we leverage these datasets to predict global health trends and disease outbreaks? The answer lies in public health data.
Public health datasets, such as the Global Health Observatory (GHO) by WHO and OECD Health Statistics, provide a wealth of data about health and diseases at a global level. For instance, the GHO offers an extensive range of datasets from 194 countries on multiple health topics, serving as a crucial resource for predicting global health trends and disease outbreaks.
On a more national level, there are several datasets that can be useful for machine learning models focusing on healthcare trends and system analysis. Some examples include:
- OECD Health Statistics, which offer comparative statistics on health and health systems across OECD countries
- Chronic Disease Data from the US CDC, which provides information on chronic conditions
- OECD Hospital Performance dataset, which can be used to develop predictive models and personalized treatment approaches
These datasets can provide valuable insights for those who analyze data, focusing on healthcare trends and improving healthcare systems.
National and global health statistics
In the realm of national and global health statistics, sources like WHO GHO and OECD Health Statistics offer a wealth of information. The WHO Global Health Observatory (GHO), for instance, offers extensive health-related datasets and reports from 194 countries, covering topics from mortality and nutrition to health systems and specific diseases.
On the other hand, OECD Health Statistics 2023 provides comprehensive health and health system statistics across countries in the Organisation for Economic Co-operation and Development (OECD). Additionally, databases like the SEER Cancer Incidence from the US National Cancer Institute contain detailed information on cancer cases, including data related to race, gender, and age. These platforms provide crucial data for machine learning models to analyze healthcare trends and system analysis at a national level.
Specialized datasets for chronic conditions
Chronic conditions, such as heart disease and diabetes, are a major burden on healthcare systems worldwide. Specialized datasets for these conditions offer a unique opportunity for machine learning applications. The Chronic Disease Data from the US CDC, for example, provides 124 indicators of chronic disease data from various states and territories, instrumental in analyzing and understanding chronic conditions at a large scale.
The OECD Hospital Performance dataset includes national and hospital-level data on 30-day mortality after acute myocardial infarction, a key metric for heart disease research. The CHDS datasets examine intergenerational health and disease, contemplating genetic, social, personal, and environmental factors, yielding insights critical for chronic disease management and intervention strategies.
The Big Cities Health Inventory Data Platform amasses data across 120 health metrics, including chronic conditions, from 35 large US cities, enhancing the understanding of urban health dynamics.
Bridging biomedical imaging and machine learning
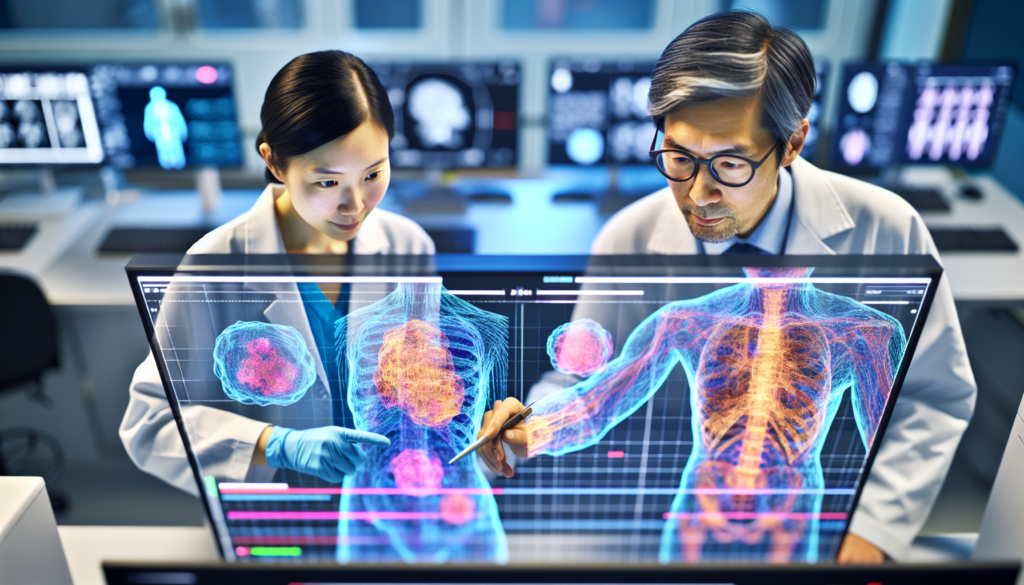
Venturing further into the healthcare data landscape, we discover a connection between two crucial and complex fields – biomedical imaging and machine learning. Biomedical imaging datasets are instrumental for AI in cancer detection, providing vital data for machine learning to:
- accurately predict or classify health outcomes
- identify patterns and anomalies in medical images
- assist in diagnosis and treatment planning
- improve the efficiency and accuracy of medical imaging techniques
This integration of biomedical imaging and machine learning has the potential to revolutionize healthcare and improve patient outcomes.
Deep learning, notably convolutional neural networks (CNNs), has shown high effectiveness in medical imaging, aiding in crucial aspects like:
- diagnosis
- disease characteristics
- stratification
- treatment response in cancer
For example, a deep-learning model trained on tens of thousands of mammogram images was successful in identifying malignancy in biopsies, demonstrating its potential to support radiologists in breast cancer detection.
Machine learning models, such as the one developed by MIT researchers, can potentially surpass traditional risk assessments by predicting breast cancer years in advance using mammogram images. These practical applications of healthcare datasets highlight the potential of machine learning in improving patient outcomes and saving lives.
The impact of TCIA data on AI development
Among the various biomedical imaging datasets, the Cancer Imaging Archive (TCIA) stands out for its impact on AI development in the study of cancer. The TCIA is a comprehensive genomics database that provides crucial data for AI development.
AI utilizes TCIA data in radiogenomics research to find links between imaging features and genetic information, enhancing the prediction of treatment side effects. Datasets from the Broad Institute Cancer Program amplify AI cancer research by offering varied tumor, cell, and gene expression information, enabling the development of targeted cancer therapies.
Advancements through child health imaging studies
While most healthcare datasets focus on adult populations, there’s a growing recognition of the importance of pediatric-specific datasets. The Child Health Imaging Studies are dedicated to pediatrics and aid in forming machine learning solutions that cater to the unique dataset needs of pediatric populations, such as different growth and development compared to adults.
Machine learning applications in pediatric imaging include:
- Bone age assessment in children
- Predicting various developmental disorders
- Improvements in imaging workflows
- Detection of imaging artifacts
- Automated diagnosing of injuries
- Treatment strategies for childhood cancers
These advancements in machine learning are shaping pediatric imaging and diagnosis.
Medical text and natural language processing
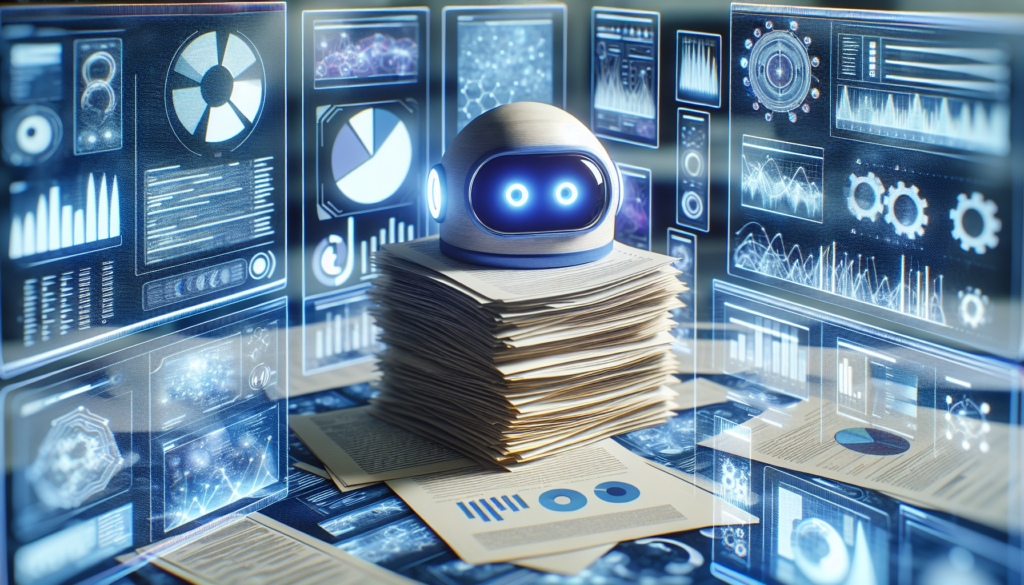
Continuing our exploration, we encounter another critical element within the healthcare data landscape – medical text. Clinical text often contains specialized medical jargon and acronyms, requiring specialized NLP models for accurate extraction and understanding of medical information.
NLP techniques for extracting information from clinical text include rule-based methods, statistical techniques using machine learning, and employing transfer learning. But how exactly does this work? And what are some of the practical applications of NLP in healthcare?
Mining insights from clinical notes
Clinical notes are a treasure trove of clinical data, offering insights into a patient’s:
- history
- symptoms
- diagnosis
- treatment
Specific NLP techniques are employed to transform raw clinical notes into structured information that can be utilized for machine learning.
Rule-based systems, decision trees, and neural networks are among the models used in NLP for pattern identification and information extraction from clinical notes. Dedicated libraries and models such as spaCy, ScispaCy, BioBERT, ClinicalBERT, and Med7 are specifically designed to process medical-related language found in clinical notes.
An AI platform by IBM Watson Health analyzes medical records with both structured and unstructured data to recommend personalized cancer treatment, demonstrating the practical use of NLP in healthcare.
Harnessing scientific literature for AI training
Scientific literature, such as published medical research articles and abstracts from databases like PubMed and CINAHL, is another rich source of data for AI training. Large language models (LLMs) such as GPT-3 and BERT, trained on extensive datasets, can perform a wide range of NLP tasks, including:
- Text summarization
- Entity recognition
- Sentiment analysis
- Question answering
These models can process scientific literature with high accuracy, making them valuable tools for the scientific community, researchers, and scientists.
Transfer learning, which involves taking a machine learning model pre-trained on vast corpuses of text and fine-tuning it for specific tasks in healthcare, is another effective technique for processing scientific literature. These techniques allow AI models to harness the wealth of knowledge contained within scientific literature, further enhancing their ability to make accurate predictions and recommendations in healthcare.
Privacy-preserving techniques in healthcare data
Venturing further into the healthcare data landscape, we must remember that this treasure trove contains not just data, but sensitive personal information of individuals. Healthcare data is inherently sensitive as it contains confidential information about individuals. Protected Health Information (PHI) under HIPAA includes identifiers such as:
- names
- dates
- contact information
- social security and account numbers
- full-face photos
- similar details
When preparing healthcare datasets for machine learning, additional steps are required to ensure compliance with privacy regulations like HIPAA. This is where privacy-preserving techniques like anonymization and de-identification come into play. But how exactly do these techniques work? And how can they maintain the utility of data for machine learning while preserving privacy?
Anonymization and de-identification strategies
Anonymization and de-identification are two key methods used to prepare medical data for research or business while preserving patient privacy. Anonymization is the process that permanently severs the link between data values and data subjects, although some argue it is never fully irreversible.
On the other hand, de-identification is designed to protect patient privacy by:
- altering, deleting, or limiting data elements, sometimes in a reversible way for future re-association
- using advanced techniques such as k-anonymity and differential privacy to offer enhanced assurances of safeguarding healthcare data
- employing methods like synthetic data generation and data minimization strategies to preserve privacy while maintaining data utility.
Balancing data utility and confidentiality
Balancing data utility and confidentiality is a challenging but necessary part of using healthcare data for machine learning. Ethical considerations in utilizing patient data, such as privacy and informed consent, are imperative in protecting individual rights.
Data anonymization, de-identification, and minimization are key strategies employed to safeguard privacy while minimizing risks to data subjects. The use of synthetic data not only protects privacy but can also help alleviate biases in datasets, making them more representative and useful for research.
With healthcare data breaches posing an annual financial burden estimated at $4 billion, protecting data through balanced utility and privacy measures is critical for the financial and reputational health of the industry.
Navigating access to healthcare datasets
After examining various types of healthcare datasets and their machine learning applications, a crucial question arises – how can we access these datasets? Open-source repositories and data-sharing platforms facilitate the availability of diverse healthcare datasets essential for machine learning development.
Notable healthcare datasets include:
- The journal-published Kent Ridge Biomedical Datasets
- OpenFDA with drug-related data
- Varied medical datasets from the DHS Program
- Medicare’s comprehensive healthcare information
Platforms like Vivli serve to coordinate the sharing and scientific use of clinical research data, providing access to a range of global healthcare data from individual country surveys to cross-country comparisons.
But accessing specific datasets on platforms like Vivli requires several steps:
- Searching the study database
- Submitting a data request form
- Gaining approval
- Agreeing to a data use agreement
So, how can we streamline this process? And what other sources of healthcare datasets are available?
Open data initiatives and repositories
Official sources like Data.gov.au and HealthData.gov serve as the official sources of Australian and U.S. government open data, respectively, providing valuable healthcare datasets for research. Medicare.gov and the CDC WONDER database complement these resources with specialized datasets such as Drug and Health Plan Data and public health information on a broad range of healthcare topics.
Non-profit open data repositories such as TCIA contribute to the field by capturing and disseminating high-quality, curated life science dataset crucial for training and validating machine learning models. Internationally, resources like Japan’s Life Science Database Archive and the OpenFDA platform extend the range of available open data, giving researchers access to datasets about organs, antigens, chemicals, and FDA data.
Collaborations with healthcare providers and pharma
While open data initiatives and repositories offer an abundant source of healthcare datasets, collaborations with healthcare providers and pharmaceutical companies provide another avenue for accessing valuable data. For instance, Google is actively inviting collaborations with healthcare providers and pharmaceutical companies on large medical datasets to further healthcare research.
These collaborations not only provide access to vast amounts of healthcare data but also pave the way for combined efforts in tackling healthcare challenges. By working together, tech companies, healthcare providers, and pharmaceutical companies can leverage their respective strengths to make significant strides in healthcare research and machine learning development.
Practical applications of healthcare datasets in ML
Having explored the expansive healthcare data landscape and the tools employed in its exploration, it’s now time to examine the bountiful insights we’ve unearthed. Real-world examples of machine learning applications in healthcare include:
- Predictive analytics for early detection of diseases
- Personalized treatment plans based on patient data
- Fraud detection in healthcare billing
- Medical image analysis for diagnosis and treatment planning
- Drug discovery and development
These examples highlight the potential impact of healthcare datasets on patient care and treatment outcomes.
Sheba hospital, for instance, utilized MLOps to deploy real-time AI services. Machine learning models anticipated sepsis in ICU patients and predicted acute kidney injury, showcasing the value of healthcare datasets for predictive analytics. Data gleaned from these specialized datasets enable the creation of predictive models and personalized treatment approaches, addressing the complexities of chronic conditions like diabetes and heart disease.
Case studies in disease prediction and management
Machine learning models have made significant strides in disease prediction and management. For example, datasets such as the California Surgical Site Infections and All-Cause Unplanned 30-Day Hospital Readmission Rate have been used for researching healthcare-associated infections and understanding healthcare quality.
The Outcomes and Assessment Information Set (OASIS) assists in measuring and improving the quality of home health services using healthcare data. Partnerships such as Google’s collaboration with healthcare institutions have enabled the exploration of how machine learning can improve patient outcomes and save lives.
Innovations in personalized treatment plans
Healthcare datasets have also paved the way for innovations in personalized treatment plans. AI enhances personalized treatment plans by taking into account clinical, genomic, and social determinants of health; it facilitates therapy planning, risk prediction, and accurate diagnosis.
Precision medicine is propelled by AI-driven genomic profiling, which identifies optimal targeted therapy plans, especially for conditions like breast or lung cancer. Synthetic data generators, such as those by MOSTLY AI, can enhance machine learning models’ proficiency in early identification of patient responses to treatments.
Machine learning algorithms, like the Personalized Learning System for Warfarin Dose Prediction, are pivotal in determining ideal medication doses, thereby reducing the occurrence of harmful side effects.
Summary
As we conclude our journey through the healthcare data goldmine, it’s evident that the treasures we’ve uncovered – the vast variety of healthcare datasets and their application in machine learning – hold great promise for the future of healthcare. From electronic health records to imaging data, from genomic datasets to public health data, each dataset holds a piece of the puzzle that is healthcare. And with machine learning, we have the tools to put these pieces together, paving the way for improved patient outcomes, personalized treatments, and a deeper understanding of health and disease. As we continue to mine this goldmine, the future of healthcare looks bright with the promise of innovation and discovery.
Frequently Asked Questions
What is an example of a data set in healthcare?
One example of a data set in healthcare is the Uniform Hospital Discharge Data Set (UHDDS), which was first implemented in 1974 and has undergone several revisions.
How can AI contribute to early disease detection in healthcare data analysis?
AI can contribute to early disease detection by analyzing large sets of data from multiple sources and identifying patterns that may go unnoticed by human doctors, such as from medical images, lab results, and patient history. This can lead to early disease detection and a reduction in misdiagnosis.
What data does AI use in healthcare?
AI in healthcare uses data such as medical images (X-rays, MRIs) to analyze and diagnose diseases with greater accuracy and speed than human radiologists, aiding in early disease detection.
How is privacy preserved when using healthcare data for machine learning?
Healthcare data for machine learning preserves privacy through techniques like anonymization, de-identification, and data minimization, which remove personally identifiable information and collect only essential data elements to reduce privacy risks. These strategies help ensure that sensitive information remains secure and confidential.