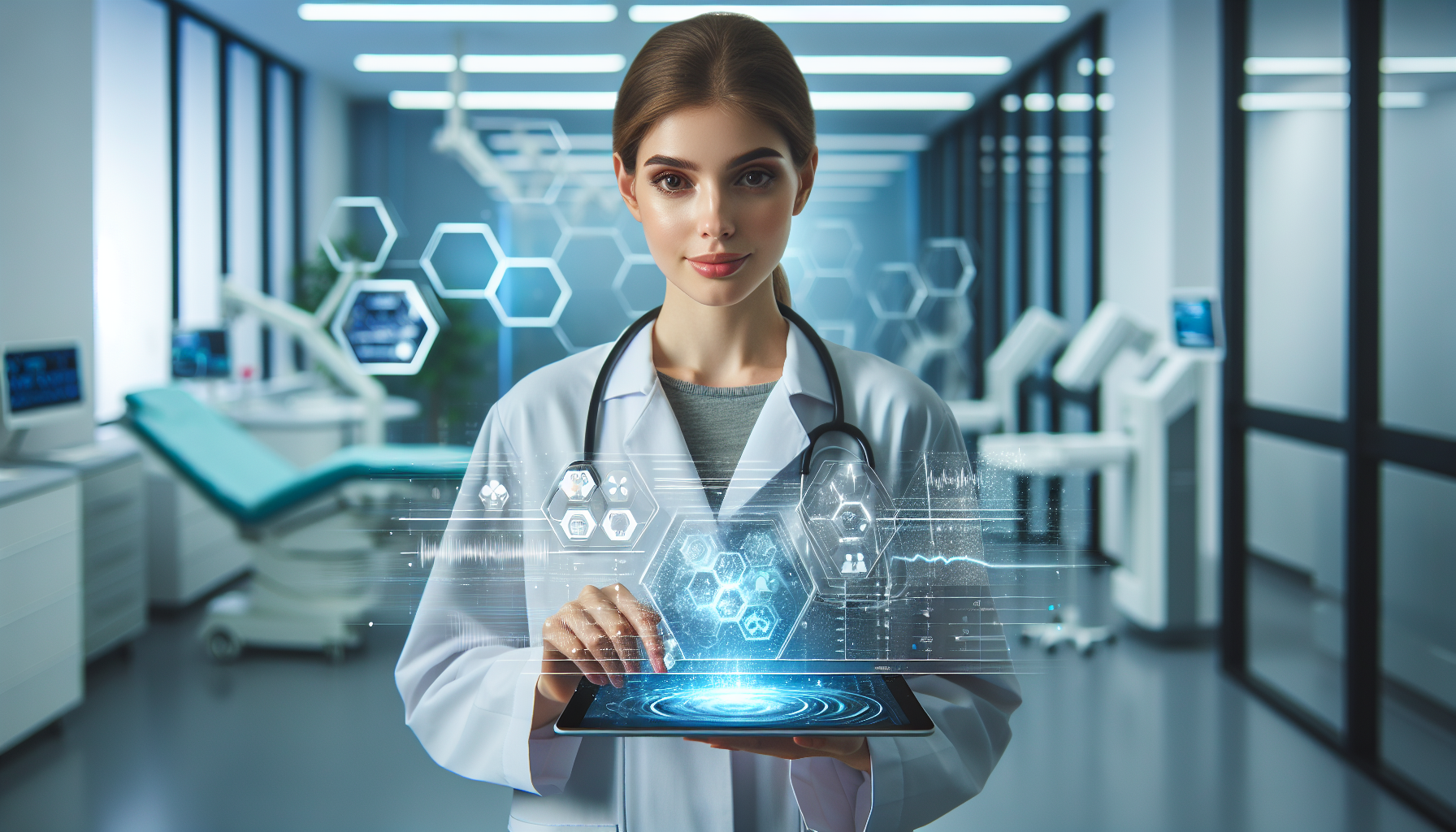
As the healthcare sector turns to technology to tackle its most pressing challenges, machine learning emerges as a critical tool, enhancing patient care and streamlining operations. But what are the practical applications of machine learning in healthcare? This article explores how machine learning use cases in healthcare are delivering personalized treatments, improving diagnostic accuracy, optimizing clinical trials, and revolutionizing drug discovery, among others, with a common goal: better patient outcomes.
Key takeaways
- Machine learning is transforming healthcare by enabling personalized medicine, enhancing diagnostic accuracy with medical image analysis, and optimizing clinical trial designs, all focused on improving patient outcomes.
- AI-driven predictive analytics contribute to early disease detection and management, especially for chronic illnesses, by analyzing data patterns to anticipate health issues and optimize treatment efficacy.
- Artificial intelligence streamlines healthcare operations by improving the efficiency of administrative processes, enhancing electronic health records, and aiding resource allocation to ensure high-quality patient care.
Harnessing machine learning for enhanced patient care
In the realm of patient care, machine learning stands as a beacon of hope, unlocking the mysteries hidden within vast arrays of patient data. Healthcare professionals are now equipped with the power to analyze medical data like never before, ushering in an era of precision medicine and personalized treatment that was once the stuff of science fiction. Hospitals and healthcare organizations are beginning to harness machine learning algorithms to not only provide better care but to enhance the very fabric of medical practice.
Machine learning is revolutionizing medical research and improving patient outcomes in several ways:
- It can sift through electronic health records (EHRs) and make sense of complex medical images.
- It contributes to patient engagement, safety, and the effective management of healthcare data.
- It paves the way for a higher standard of patient care.
Precision medicine and treatment personalization
Gone are the days of a one-size-fits-all approach to medicine. Precision medicine, fueled by machine learning, ushers in a tailored healthcare experience where treatments and prevention strategies are finely tuned to the individual’s genetic profile, lifestyle, and environmental factors,. Data scientists and healthcare professionals collaborate, wielding AI’s predictive power to analyze patient data, decipher genetic codes, and provide highly personalized treatment plans that improve patient outcomes.
This personalized approach is not only more effective but also minimizes medical errors and side effects, ensuring patient safety and care that is as unique as the patients themselves.
Medical image interpretation
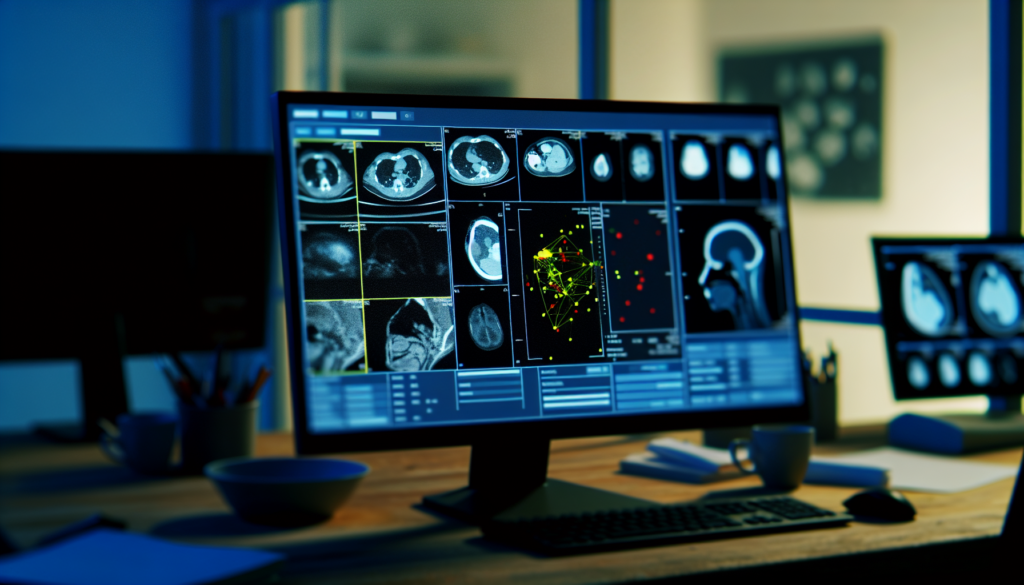
Imagine the ability to spot the earliest signs of disease, hidden within the complex patterns of medical images. Machine learning engineers are transforming medical image analysis with deep learning algorithms, enhancing the precision of diagnoses and enabling healthcare providers to act swiftly and effectively. These advanced machine learning models can:
- Analyze medical images
- Identify anomalies and pathologies that the human eye might miss
- Lead to early detection
- Significantly improve patient health outcomes.
Through AI-assisted radiology, medical professionals can now navigate the intricate visual representations of X-rays, MRIs, and ultrasounds with enhanced clarity, reshaping medical imaging and clinical practice.
Optimized clinical trial design
In the quest to bring new and effective treatments to patients faster, machine learning is optimizing the design and execution of clinical trials. By analyzing historical data and patient records, AI/ML-based predictive analytics can forecast patient recruitment, monitor trials in real-time, and ensure patient safety, thus reducing the time and cost associated with drug development.
This streamlining of the clinical trial process not only accelerates the availability of new drugs but also enhances the efficacy of treatments, ensuring that personalized treatment plans reach those who need them most.
Innovations in disease prediction and management
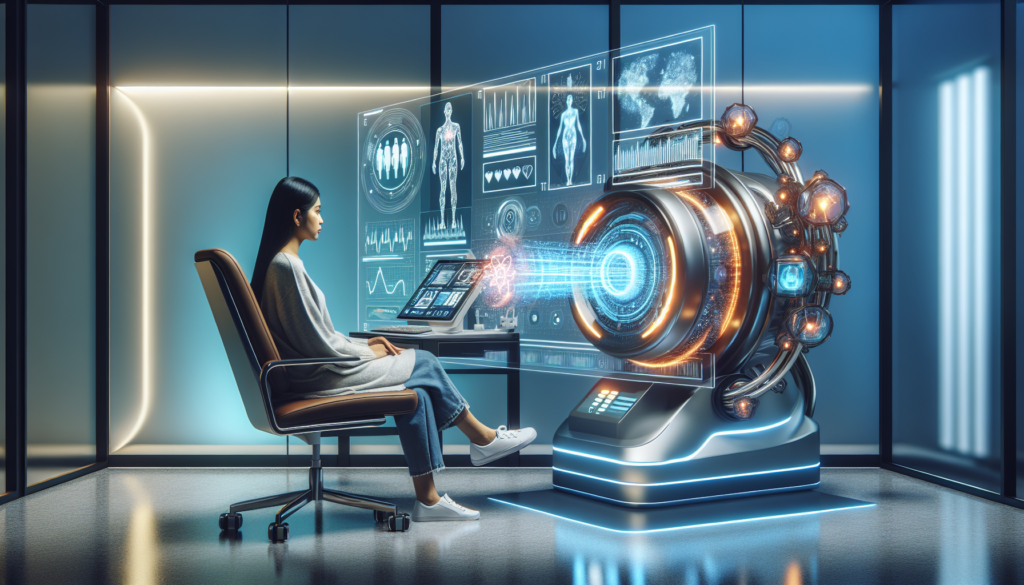
The power of machine learning extends beyond individual patient care into the broader arena of disease prediction and management. Healthcare machine learning is now a pivotal tool in:
- the early stages of disease detection
- analyzing complex healthcare data to predict the spread of infectious diseases
- identifying at-risk individuals before symptoms appear.
The integration of AI into healthcare systems enables providers to anticipate health deterioration and intervene proactively, transforming the management of chronic diseases and reducing hospital readmissions. This results in real-world evidence-based care that is both responsive and preventive, a true testament to the paradigm shift towards enhancing patient outcomes.
Predictive analytics for early detection
With the advent of machine learning technology, the healthcare industry is poised to detect diseases at their early stages, when they are most treatable. Predictive analytics, a cornerstone of healthcare artificial intelligence, sifts through historical data and patient records to flag potential health issues before they escalate. Machine learning algorithms can analyze patterns in patient data, predicting conditions such as heart failure, potentially life-threatening events like strokes, and even forecast the spread of epidemics like COVID-19, showcasing the technology’s crucial role in public health and early detection.
Chronic disease monitoring and management
As the global burden of chronic diseases grows, machine learning models stand at the forefront of a proactive approach to health management. AI-driven systems utilize real-time monitoring and analysis of health data to:
- Detect early signs of chronic conditions such as heart disease and diabetes
- Empower healthcare providers to tailor personalized care plans
- Ensure patient engagement
Through the continuous remote patient monitoring facilitated by AI, the healthcare sector can intervene promptly upon detecting shifts in a patient’s health, which not only enhances patient outcomes but also fosters a culture of proactive self-care among patients.
Streamlining healthcare operations with AI
Artificial intelligence is revolutionizing the operational side of healthcare, introducing efficiencies that directly impact patient care. Machine learning algorithms refine the administrative processes, from appointment scheduling to insurance claim processing, allowing healthcare professionals to dedicate more time to direct patient care.
AI systems, integrated within healthcare institutions, can provide the following benefits:
- Automate routine tasks
- Help manage the tsunami of data generated daily
- Lead to more informed clinical decision-making
- Improve patient safety
- Significantly reduce data-based errors
Enhancing electronic health record systems
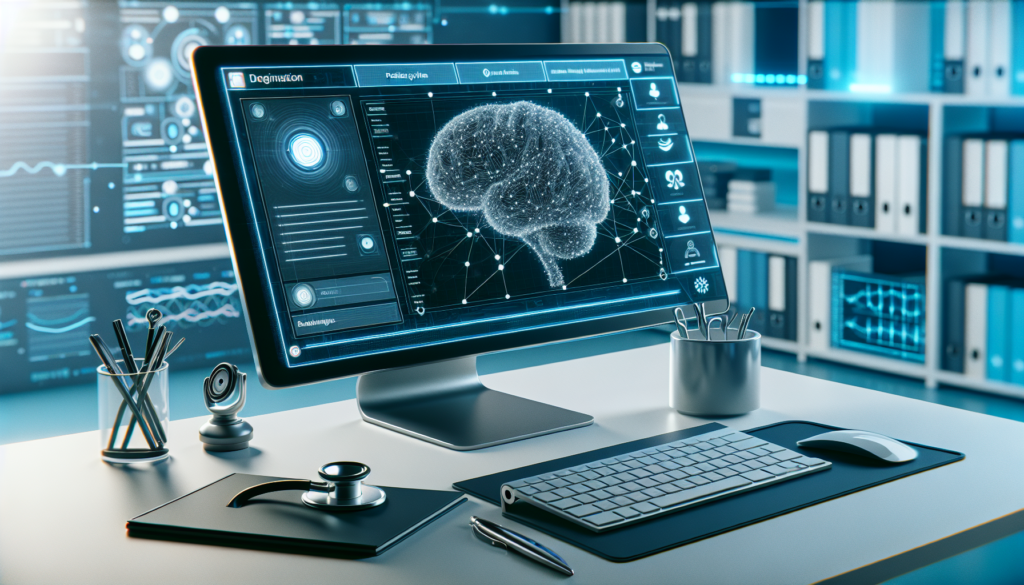
Machine learning is reshaping the backbone of healthcare information systems: the Electronic Health Record (EHR). By automating data entry and management, machine learning technology increases the accuracy and accessibility of patient records, supporting healthcare data analysis and management.
The integration of AI-driven clinical decision support systems provides real-time insights and recommendations, optimizing clinical workflows and contributing to the goal of improving patient outcomes. This technological advancement ensures that EHR systems are not static repositories of information but dynamic tools that adapt and evolve with medical practice.
Resource allocation and hospital workflow optimization
Efficiency in healthcare goes beyond the clinic and into the operational machinery of hospitals. Machine learning in healthcare provides predictive insights into patient influx, enabling the optimal allocation of resources and reducing wait times. AI aids healthcare providers by streamlining patient appointment scheduling and coordinating care, improving the patient experience.
Predictive maintenance of medical equipment, guided by AI, not only ensures uninterrupted patient care but also contributes to cost savings, reflecting machine learning’s role in enhancing operational efficiency.
The role of natural language processing in patient records
Natural Language Processing (NLP) stands as a pillar in the modern healthcare framework, bridging the gap between the intricacies of human language and the precision of machine understanding. In the context of patient records, NLP serves as the interpreter, transforming the free-text information recorded by physicians into structured, analyzable data. This transformation is vital for the efficient management of healthcare data and for enabling seamless communication among healthcare professionals,.
Clinical language understanding
Clinical Language Understanding, a specialized application of NLP, decodes the myriad medical terminologies and abbreviations that are the lifeblood of patient records. By discerning the context and nuances of clinical data, NLP systems improve the accuracy of information interpretation, which is crucial for informed clinical decision-making and patient safety.
Enhancing communication through automated documentation
In the digital age, automated documentation powered by NLP is a boon to clinicians, enabling them to spend less time on paperwork and more time with patients. Speech recognition technology, a key NLP application, captures spoken language and translates it into written text with remarkable accuracy, ensuring that patient records are detailed and precise.
This automation not only enhances patient engagement but also improves the quality of data in electronic health records, ultimately leading to improved patient care.
Improving patient outcomes through data-driven decisions
The ultimate goal of implementing machine learning in healthcare is to improve patient outcomes. Data-driven decisions, informed by machine learning models, are at the forefront of this quest. By leveraging the power of healthcare data, machine learning aids in the early detection of serious illnesses and the identification of high-risk patients, leading to timely interventions and better health outcomes.
Machine learning in diagnostic accuracy
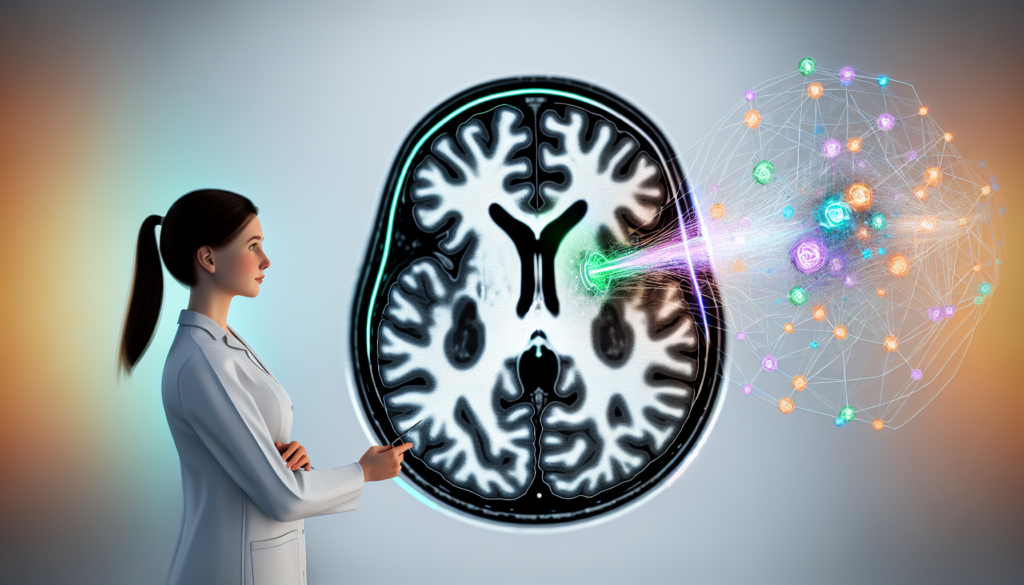
When it comes to diagnostic accuracy, machine learning is not just enhancing but redefining the standards of care. AI-powered tools analyze medical data, from ECG readings to voice patterns, to detect diseases such as arrhythmias and Parkinson’s at an early stage. These sophisticated AI models provide healthcare professionals with invaluable support in the diagnostic process, leading to improved patient outcomes and a higher standard of medical practice.
Treatment efficacy analysis
Artificial intelligence doesn’t stop at diagnosis; it extends its reach to the analysis and optimization of treatment efficacy. By evaluating treatment effects on patient cohorts and predicting individual responses, AI tools personalize healthcare, ensuring that each patient receives the most effective treatment possible. This level of analysis is pivotal in the development of evidence-based treatment guidelines that are tailored to patient needs, contributing to the overall enhancement of healthcare outcomes.
Revolutionizing drug discovery and development
Machine learning is igniting a revolution in the field of drug discovery and development, providing pharmaceutical companies with groundbreaking tools to accelerate the identification of new drug candidates and enhance the drug development pipeline. By analyzing vast machine learning healthcare datasets, algorithms are identifying promising compounds and predicting their efficacy and side effects, significantly reducing the time and resources needed to bring new drugs to market.
Accelerating the identification of drug candidates
The initial stages of drug discovery are witnessing an unprecedented acceleration thanks to machine learning. By automating the screening of compounds and employing sophisticated algorithms, machine learning is improving the speed and efficiency of the drug discovery process. This rapid identification of potential drugs expedites the development of new treatments, making a significant impact on the healthcare sector and patient care.
Enhancing the drug development pipeline
Beyond discovery, machine learning is enhancing the entire drug development pipeline, from predicting drug-protein interactions to assessing safety biomarkers. Deep learning techniques are being deployed to improve the prediction of drug efficacy and side effects, ensuring that the drugs reaching the market are both safe and effective.
The integration of AI into drug development is reshaping the pharmaceutical industry, leading to more efficient processes and better treatments for patients.
Empowering patients and providers with virtual assistance
The integration of virtual assistance into healthcare is empowering both patients and providers by offering:
- Instant, AI-driven support for routine inquiries and patient education
- Medication reminders
- Lifestyle recommendations
- Administrative support
These virtual assistants greatly enhance the efficiency of healthcare operations and patient engagement.
Virtual nursing assistants for routine inquiries
Virtual nursing assistants are alleviating the burden on human nurses by taking over routine tasks such as:
- collecting basic health information
- monitoring vital signs
- reminding patients to take medication
- answering common patient questions
This not only prevents nurse burnout but also allows nurses to concentrate on more critical care tasks, improving the quality of patient care and enhancing patient safety.
Interactive chatbots for patient education
Interactive AI-driven chatbots are transforming patient education by providing personalized and engaging interactions. By analyzing patient data, chatbots deliver tailored education and self-care advice, which is critical for chronic disease management.
Moreover, their ability to manage high volumes of interactions concurrently significantly improves the operational efficiency of healthcare providers.
Summary
As we reflect on the transformative journey of machine learning in healthcare, it’s clear that the integration of AI technologies has begun to reshape the landscape of patient care, disease prediction, drug discovery, and healthcare operations. From the personalization of treatments to the optimization of clinical trials, and from the enhancement of diagnostic accuracy to the streamlining of healthcare workflows, machine learning is improving patient outcomes at every turn. It empowers healthcare professionals with data-driven insights, enabling a more proactive, predictive, and personalized approach to healthcare. The innovations we’ve explored are not distant possibilities; they are current realities improving the lives of patients worldwide, signaling the dawn of a new era in healthcare—one that is smarter, faster, and more patient-centric than ever before.
Frequently Asked Questions
What are 3 use cases for big data in healthcare?
Big data in healthcare is used to create value-based, patient-centric care, reduce fraud and abuse, and perform predictive analysis. These applications help in improving healthcare outcomes and efficiency.
What are the use cases of machine learning?
Machine learning has various use cases, including image recognition, customer segmentation, chatbots, recommendation engines, and healthcare advancement. It is also used in marketing, sales, cybersecurity, financial transactions, transportation, and more.
What is a use case of deep learning in healthcare?
Deep learning is used in healthcare for analyzing electronic health records, including clinical notes, test results, diagnosis, and medications, at exceptional speeds and accuracy. This can greatly improve the efficiency and quality of medical data analysis.
How is machine learning personalizing patient care?
Machine learning personalizes patient care by analyzing patient data, including genetic profiles and lifestyle factors, to create individualized treatment plans tailored to each patient’s unique needs. This facilitates better patient outcomes.
Can AI improve the accuracy of medical diagnostics?
Yes, AI can significantly improve the accuracy of medical diagnostics by analyzing medical images with advanced algorithms and enabling early disease detection, often outperforming traditional methods.