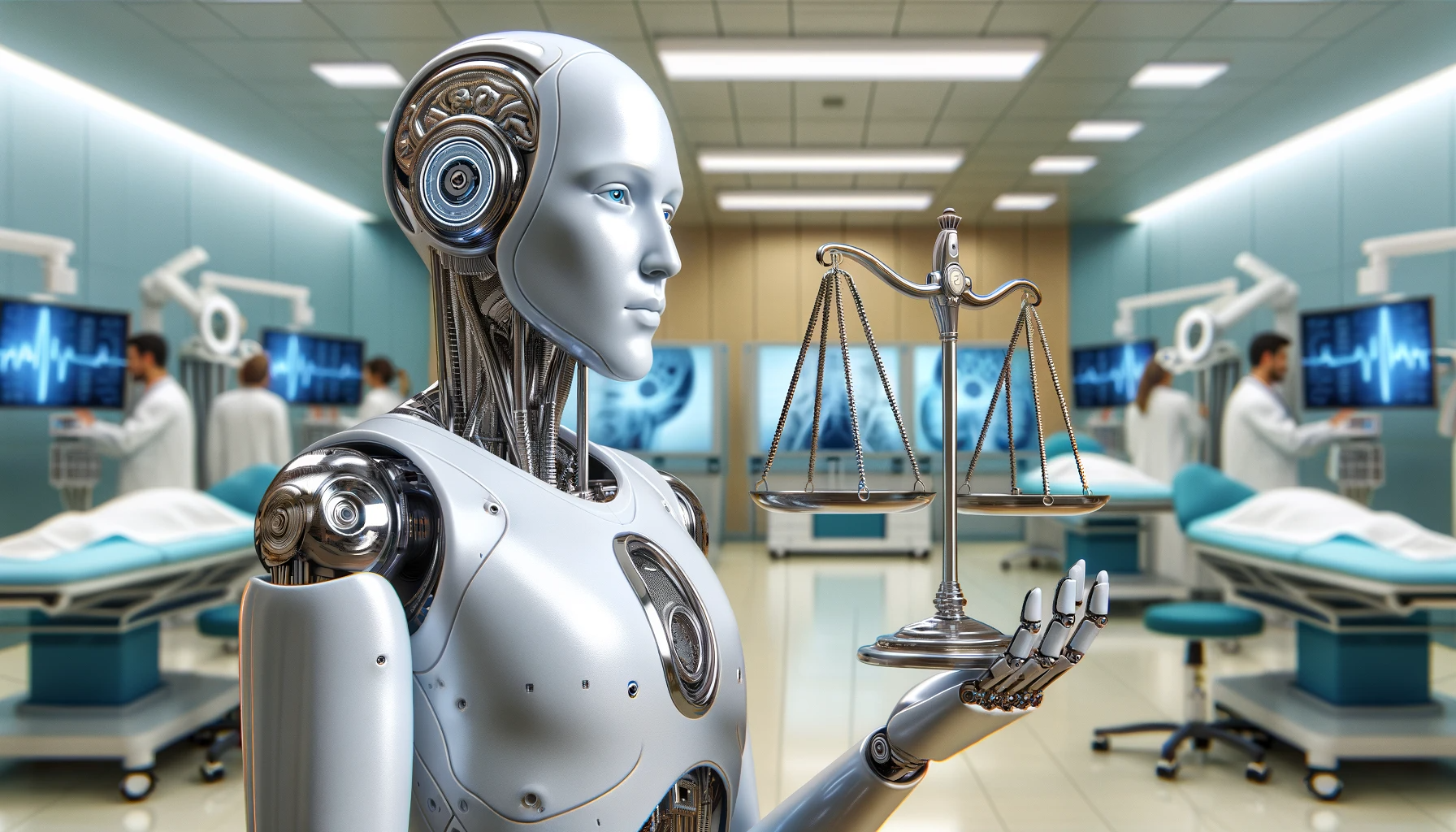
Ensuring patient safety and data protection is at the forefront as AI gains ground in healthcare. With a sharp focus, this article addresses the foremost risks of AI in healthcare – data security breaches, ethical controversies, and risk of systematic biases – outlining the imperative for vigilance and proactive safeguards in the digital age of medicine.
Key Takeaways
- AI healthcare integration presents significant risks such as data breaches and ethical dilemmas that need to be addressed through stringent security measures and consensus building on research methodologies.
- Legal and regulatory frameworks are struggling to keep up with the pace of AI advancement in healthcare, necessitating continual reassessment and updates to ensure ethical and safe use of AI technology.
- The future of AI in healthcare is promising, with advancements and innovations aimed at improving patient outcomes through personalized medicine and operational efficiency; however, managing risks and fostering collaborative efforts and best practices is crucial.
Risks and challenges in AI healthcare integration
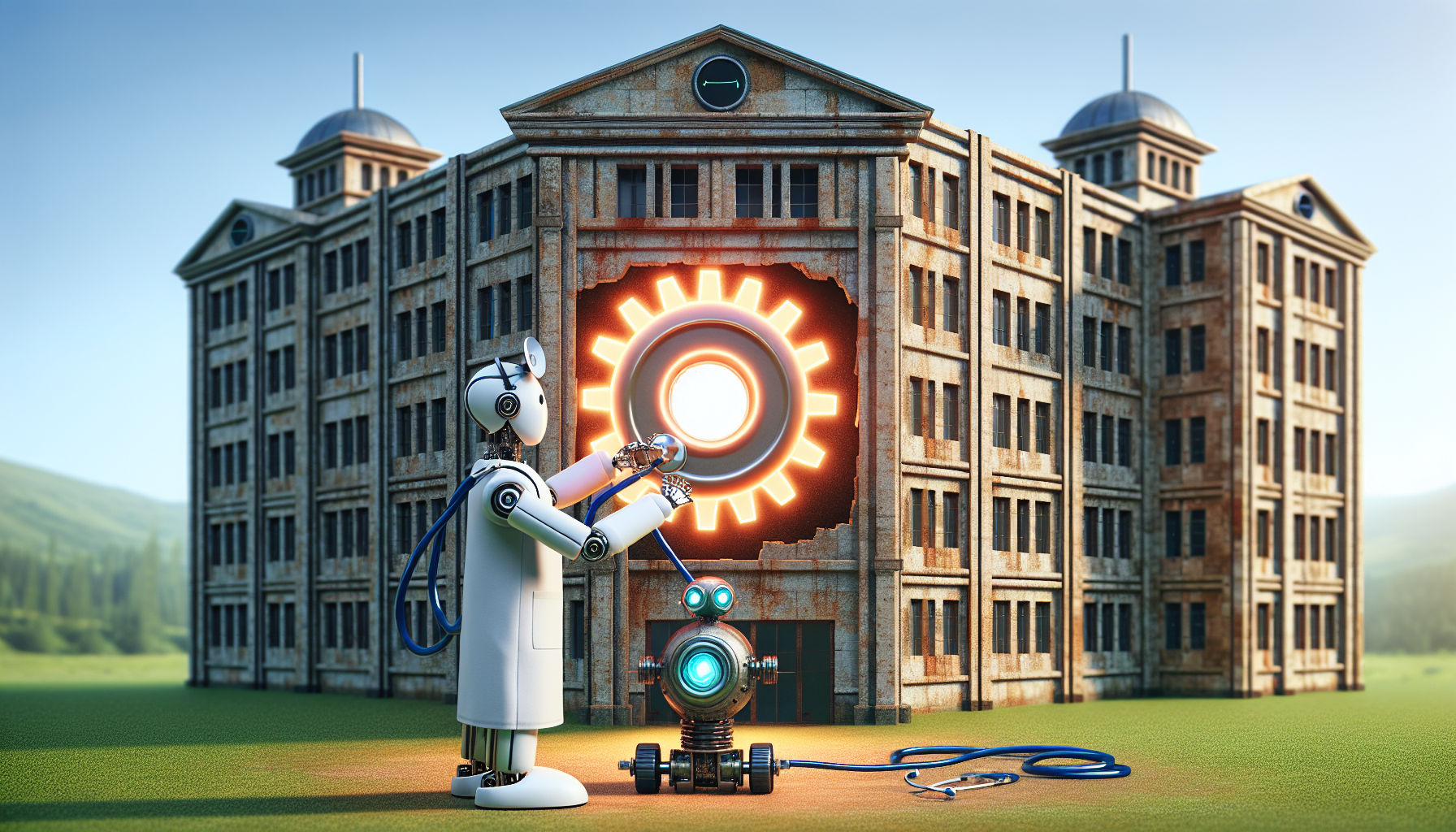
The incorporation of AI in healthcare has opened up a world of possibilities, from efficient diagnosis to personalized treatment plans. However, this journey is not without its hurdles. AI systems use vast amounts of sensitive patient data, which raises the risk for potential data leakages and breaches, highlighting the need for stringent security measures. Furthermore, the field of AI healthcare research is grappling with methodological issues, including the absence of consensus on research methodologies, leading to hurdles in validating AI tools.
The rapid advancement of AI technologies in healthcare has also led to new ethical dilemmas. Decisions must align with the ethical principles of patient care. Hence, navigating these challenges is key to fostering trust and ensuring the successful adoption of AI in healthcare, as we strive towards its integration.
Data privacy and security issues
The use of AI in the healthcare field brings to light the utmost importance of maintaining data privacy and security. The confidential information used, known as Personally Identifiable Information (PII), is protected under strict laws such as GDPR and HIPAA, highlighting how crucial it is to safeguard patient data within this realm. With an increase in demand for healthcare AI adoption also comes a higher risk of potential breaches. This has already become reality with over 6 million cases reported by October 2022 solely in the United States.
Even AI systems themselves can be susceptible to security vulnerabilities and cyber-attacks which jeopardize confidentiality regarding patient data. As more private entities take ownership over these technologies within the healthcare industry, concerns are growing around protecting patients’ health-related personal information, thus emphasizing stricter measures towards preserving their privacy rights. Despite its promising capabilities within medical settings, effective management must prioritize addressing any arising issues concerning both ensuring safekeeping standards when handling sensitive patient records at stake alongside mitigating existing risks associated with utilizing Healthcare Artificial Intelligence advancements.
Ethical dilemmas
Incorporating AI into healthcare presents a set of moral dilemmas. When it comes to AI systems in the field of healthcare, being accountable and responsible is crucial, especially if they are involved in making decisions that directly impact patient care. For instance, an erroneous prediction by an AI system could result in a medical mistake, leading us to question who should be held liable, the healthcare provider or developer behind the AI technology or even the entity that owns such systems.
As we see more reliance on AI for decision-making (traditionally handled by humans), there arises a potential threat to patient autonomy. If an Ai system makes diagnoses or recommends treatments on its own accord, what happens to patients’ role when it comes down to informed choices about their health? These are pressing matters that require solutions from our ever-evolving healthcare sector as we continue integrating AI into practice within this realm.
AI implementation obstacles
Implementing AI in healthcare is not a straightforward process. A significant hurdle is the AI chasm, which arises due to clinically irrelevant performance metrics and the lack of specific implementation frameworks. This gap hinders the effective integration of AI in healthcare practices. Moreover, the rapid development of AI technology contrasted with slow healthcare implementation rates results in a mismatch between available AI capabilities and their practical use.
The barriers to the application of AI in clinical settings include:
- The absence of robust empirical data supporting AI’s effectiveness
- The need for seamless incorporation into healthcare workflows without disruptions
- The demand for modifications in healthcare professions and practices, triggering the need for new roles and interdisciplinary collaboration
Successful AI implementation requires not just technological advancements but also addressing these barriers.
AI impact on medical professionals and patient care
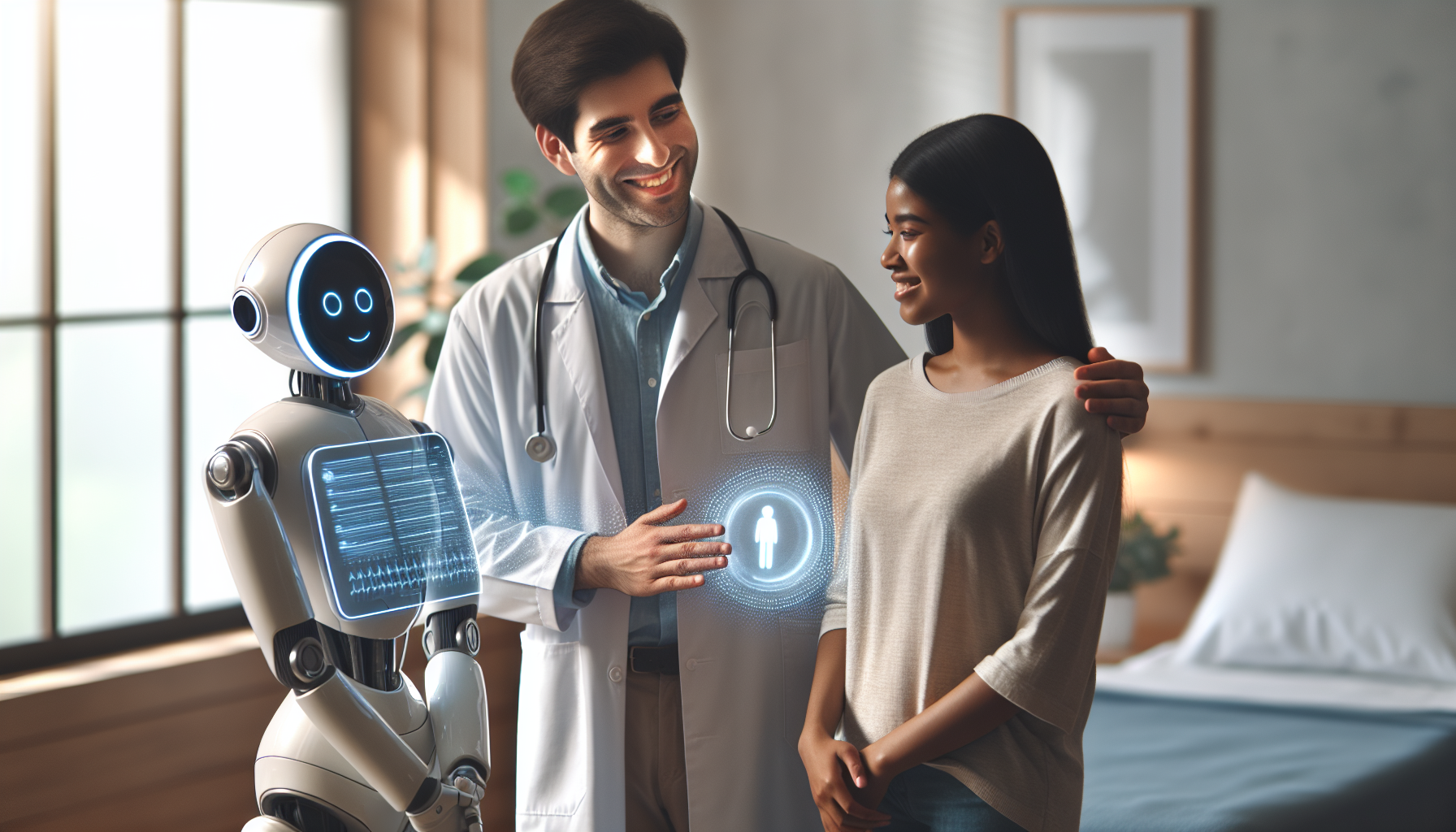
The use of artificial intelligence (AI) in healthcare has a significant and diverse impact on medical professionals, including medical practitioners, and the quality of patient care. In various areas such as diagnosis, treatment planning, drug discovery, and administrative tasks like record keeping and scheduling appointments. AI is used to analyze patient data efficiently leading to accurate results. It also helps create personalized treatments by analyzing individual information like past medical history and genetic makeup.
Moreover, AI plays an essential role in accelerating the drug development process by sifting through vast amounts of data for potential candidates. Ultimately,this not only improves overall patient care but also leads to cost savings and increases efficiency in healthcare administration.It’s important to note that while AI can provide valuable support, it cannot replace human qualities such as empathy, basic observation abilities, and compassion, which are critical factors especially when it comes to disciplines like psychiatry.
Overall, the presence of AI technology brings numerous benefits within the field of medicine, for instance, assisting doctors with more efficient diagnoses, task completion at lower costs, and making use of complex problem-solving methods.
AI encourages faster innovations, targeted health solutions reduce overheads, which serves towards promoting better delivery services, advancements in medication technologies & providing efficiencies making processes smooth and expedient.In conclusion, the continuous developments within the applied sciences will revolutionize how we approach providing patients around the globe. Developing these new technologies together contributes significantly to improving outcomes across all aspects of medically related activities. Being able to enhance concerns most involving pharmacological interventions procedures, pathways hospital logistics among others is a top priority endless possibilities more collaboration could bring forth valuable insights increasing output for future generations success rate.Precision achievements.Let us embrace new frontiers!
Medical staff job displacement concerns
The integration of artificial intelligence in healthcare has sparked debates about its impact on employment within the industry. Contrary to the common fear of job displacement, the fusion of AI with healthcare is anticipated to foster employment growth, particularly in Health Information Technology. This advancement has led to the emergence of various AI-centric roles in healthcare, including Health Data Analysts, Medical Data Scientists, Healthcare AI Engineers, and Healthcare AI Project Managers.
For professionals aspiring to thrive in the healthcare sector, adapting to these evolving AI-related job opportunities is crucial. AI technology is not aimed at replacing human roles; rather, it is designed to enhance the capabilities of knowledge workers and IT professionals. There’s a growing preference among healthcare organizations for roles focused on AI, signifying the sector’s inclination towards AI-enhanced functions. This latest trend in medical AI not only paves the way for new job creation but also enriches existing roles, all without significantly disrupting current job structures.
Dispelling initial concerns over job losses, AI in healthcare is increasingly viewed as a source of opportunity rather than a threat to existing jobs. The introduction of AI into hospitals and other medical facilities is not meant to cause unemployment. Instead, AI serves as an additional tool through which medical care can be delivered more efficiently, thereby enhancing the quality of services provided. This forward-thinking approach signals a transformative era in healthcare, where AI acts as a catalyst for both technological advancement and job growth.
Human error reduction vs. overreliance on AI
While artificial intelligence has the potential to reduce human error in healthcare, it is essential to balance this benefit against the risk of overreliance on AI. Overreliance on AI can lead to automation bias, resulting in an uncritical acceptance of AI-generated conclusions. Studies show that both experts and novices in medicine may not dismiss inaccurate advice from AI systems, highlighting the risk of automation bias.
Interface design in AI systems can either mitigate or exacerbate the problem by either encouraging or not supporting critical thinking among healthcare professionals. Incorporation of AI within clinical workflows should be crafted to foster deliberation and minimize overdependence. Hence, healthcare professionals must calibrate trust in AI systems to avoid blind acceptance and undue skepticism.
AI bias and discrimination in healthcare
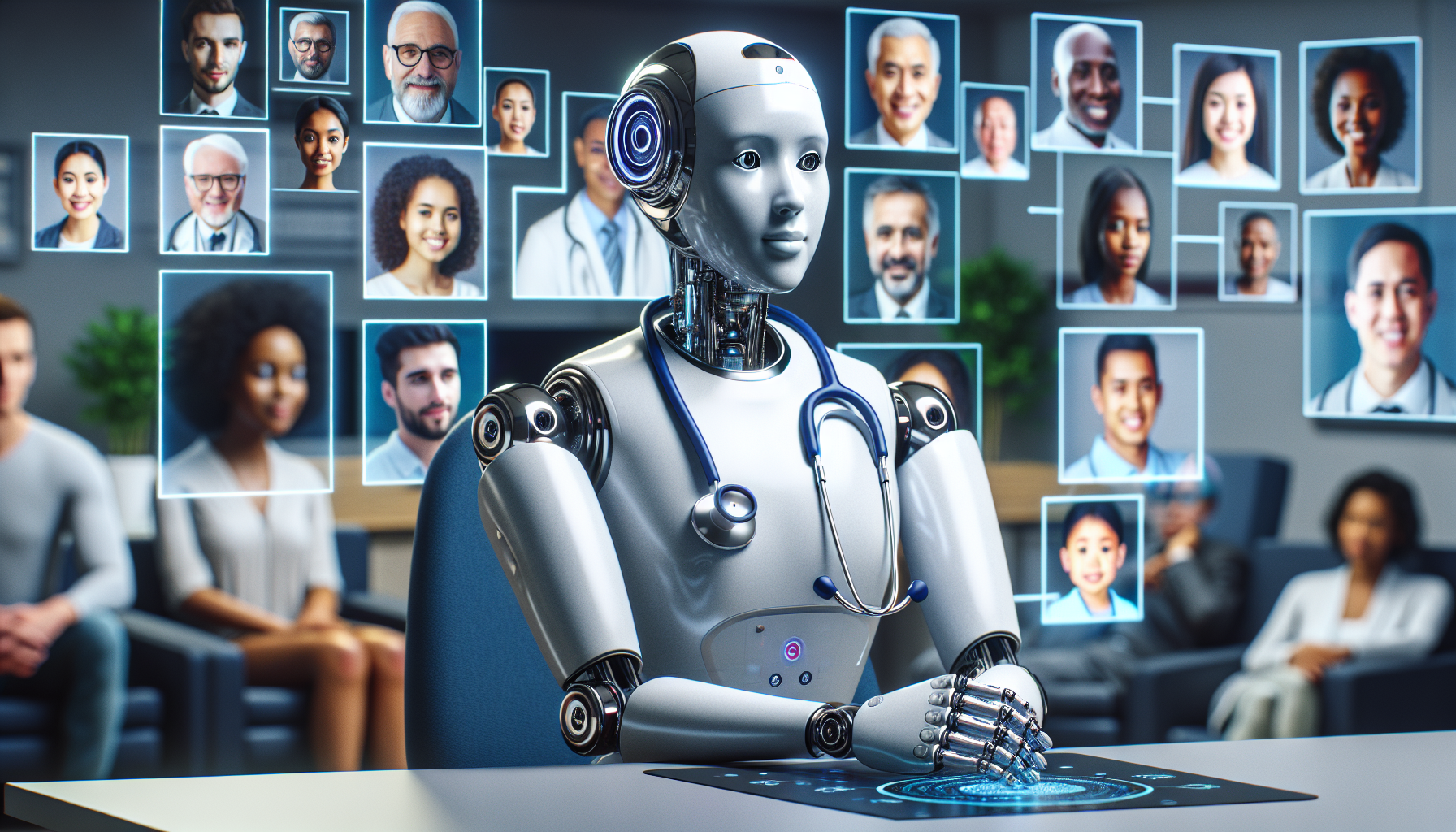
One pressing concern in healthcare is the potential for AI systems to exhibit bias and discrimination, which can have serious implications on clinical decision-making and patient care. Biased outcomes from these algorithms are often a result of skewed data or developer biases, leading to inaccurate predictions and inappropriate treatment that disproportionately affects marginalized groups.
A major challenge in addressing this issue is the inherent opacity of AI systems, making it difficult to identify and correct any existing biases – commonly referred to as the “black box” problem. As such, efforts are underway towards creating more inclusive datasets, utilizing bias-detecting algorithms during development, establishing ethical guidelines for AI use in healthcare settings, and promoting collaboration among stakeholders involved.
In order to effectively tackle issues of bias within AI systems used in healthcare contexts, it is crucial that we continue implementing measures aimed at identifying,balancing out,disclosing,reducing,the impact caused by prejudice related elements present at various stages throughout system design.The collaborative effort between all parties invested in ensuring fair practice must remain steady if significant progress toward mitigating adverse health effects on vulnerable communities via biased algorithmic decisions is ever going to be achieved.
Causes of algorithmic bias in health industry
Algorithmic bias in AI inside healthcare can appear from numerous factors, often intertwining and reinforcing each other. here are some key causes:
Data skewness | When the data used to train AI models isn’t reliable of the whole population, the AI can develop biases. as an instance, if a dataset predominantly includes records from certain age groups, races, or genders, the AI will be less accurate for underrepresented groups. |
Historical biases in information | AI models can inadvertently examine and perpetuate historical biases present in the training data. as an example, if historic data displays past inequalities in healthcare access or remedy effectiveness for certain groups, the AI might replicate these biases. |
Limited diversity in development teams | A lack of variety among the scientists and engineers who develop and broaden AI algorithms can lead to unconscious biases in how these systems are programmed to interpret information. |
Oversimplification in algorithm design | AI models may oversimplify complicated clinical situations and social contexts. This oversimplification can lead to misinterpretation of facts, especially for conditions that show up differently throughout various populations. |
Feedback loops | AI systems in healthcare regularly examine constantly from new information. If the preliminary model has biases, these may be bolstered and amplified through the years as the system translates new data via the lens of its existing biases. |
Socioeconomic factors | Disparities in healthcare access and quality because of socioeconomic factors also can be reflected within the information. AI trained on such data won’t correctly represent the needs and conditions of less privileged groups. |
Regulatory and standardization gaps | The lack of comprehensive requirements and rules specifically for AI in healthcare can result in inconsistencies in how bias is addressed across distinct systems and programs. |
Insufficient testing and validation | If AI systems aren’t thoroughly tested across diverse population groups, there is probably a failure to discover biases earlier than those systems are deployed in real-world settings. |
In order to ensure fairness and effectiveness in healthcare using AI technology, it’s necessary to tackle key factors contributing towards algorithmic biases such as diverse development teams along with unbiased training datasets gathered from various sources instead of only relying on primarily academic medical centers.Data collected through this approach will better reflect society demographics ensuring adequate representation across all categories including race,gende,rage, and socio-economic background.This step would go a long way towards improving ethical standards and credibility of AI usage in the health care field.
Consequences for patient care
The impact of AI bias on patient care can have serious consequences. Biased algorithms and models in healthcare can lead to delayed, inappropriate or harmful outcomes for patients, such as incorrect diagnoses and suboptimal treatment plans. This exacerbates existing disparities within the healthcare system by providing lower-quality care to specific populations.
Using biased AI in healthcare has the potential to erode trust among patients, especially those from marginalized groups who may feel their unique needs are being overlooked by these technologies. As a result, addressing AI bias goes beyond just a technical issue, it is crucial for ensuring quality patient care and maintaining trust in our healthcare system.
Strategies to mitigate AI bias in healthcare
In today’s fast-evolving healthcare sector, AI plays a pivotal role. However, to ensure that this technology benefits everyone equally, tackling AI bias is critical. Here’s a user-friendly guide to a comprehensive strategy:
- Embrace diversity in data. It’s crucial to train AI models on varied datasets representing the whole population, considering factors like age, gender, race, and more. Keeping these datasets current with changing demographics is also important.
- Monitor for bias. Regularly check AI algorithms for bias, using statistical methods to ensure fairness across different patient groups.
- Team up across disciplines. Collaboration is key. By bringing together data scientists, healthcare professionals, ethicists, and patients, we can better identify and address potential biases in AI.
- Focus on ethical AI. Adhering to ethical standards in AI development is non-negotiable. This means ensuring decision-making processes in AI are transparent and fair.
- Stay regulatory compliant. It’s important to not only comply with current regulations but also contribute to setting new standards for AI in healthcare, focusing on data quality and algorithm fairness.
- Educate healthcare professionals. Training healthcare workers about AI’s capabilities and limitations, especially regarding bias, is essential for its effective and conscious use.
- Put patients first. Involving patients, especially from underrepresented groups, in decision-making can provide critical insights into reducing AI bias.
- Conduct regular audit. Assess AI systems’ impact on different demographic groups regularly, and use this data to continuously improve these systems.
- Collaborate with policymakers. Working with policymakers can help create a supportive environment for unbiased AI development in healthcare.
- Cultivate inclusivity and awareness. Establishing a workplace culture that values inclusivity and is alert to biases ensures that concerns about AI bias are openly discussed and addressed.
By embracing these strategies, the healthcare industry can significantly reduce AI bias, leading to more equitable and effective healthcare solutions for everyone.
Regulatory and legal aspects of AI in healthcare
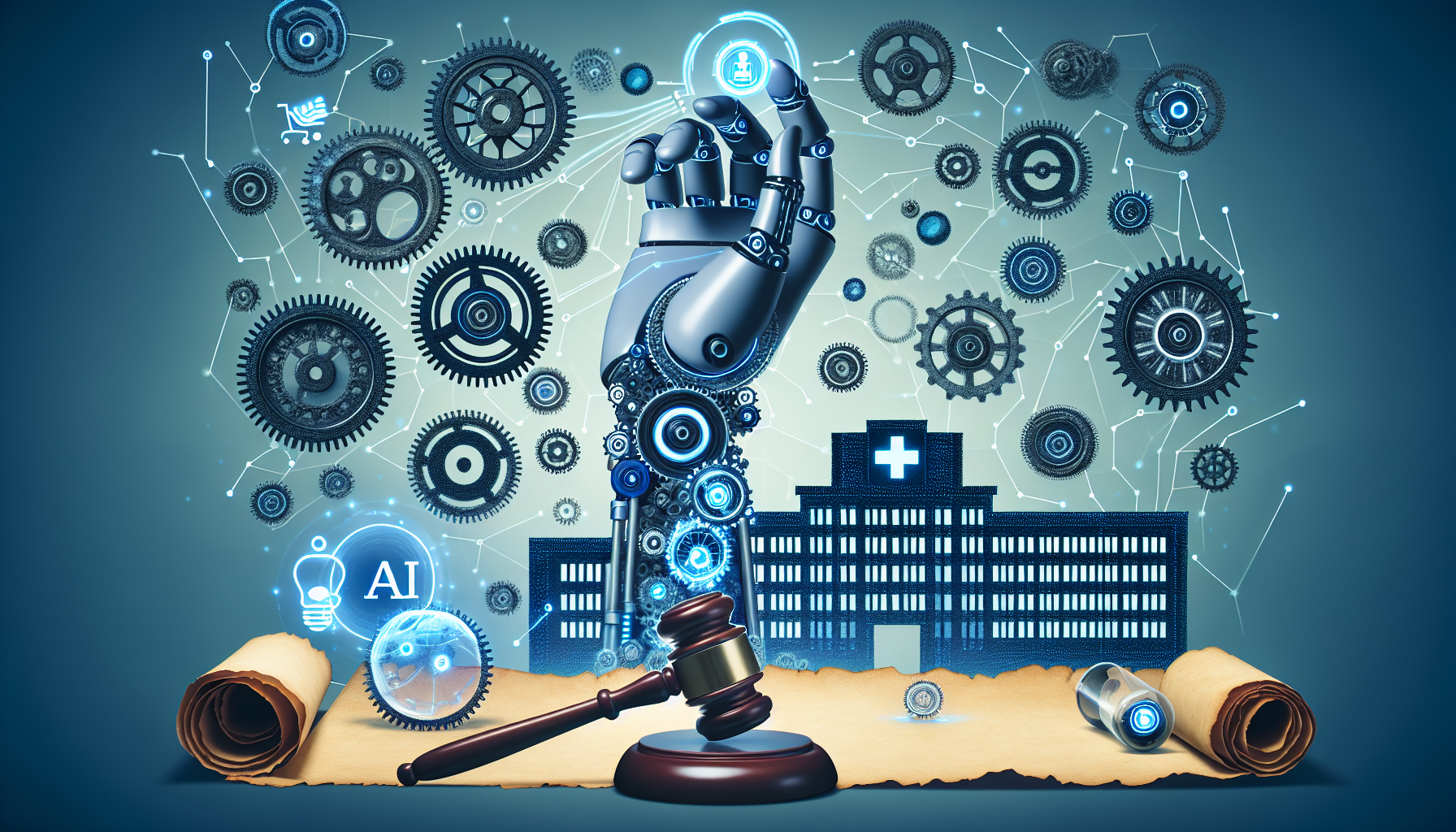
As AI technology advances at a rapid pace in the healthcare industry, existing regulatory and legal systems are struggling to keep up. There is a significant risk that these systems will fall behind as they attempt to govern ever-evolving AI technologies. In order for effective use of AI in healthcare, it is crucial that laws and policies be updated to regulate the sharing of patient information and personal data.
The regulation and oversight of AI in healthcare play a critical role in ensuring ethical, safe, and efficient use of this technology. As we continue to see integration between traditional medicine practices and advanced artificial intelligence solutions, ongoing assessment and updates must occur within regulatory structures to effectively address challenges posed by such emerging technologies.
Current regulatory landscape
In the healthcare industry, there are several important policies and guidelines that regulate the use of AI. One such policy is the FDA’s AI/ML-based Software as a Medical Device (SaMD) Action Plan which aims to safely incorporate innovative uses of AI in healthcare. This plan includes a “predetermined change control plan” for premarket submissions regarding updates or changes to algorithms, with an emphasis on transparency and risk management.
Other regulatory frameworks are also adapting to address challenges posed by advancements in AI technology within healthcare. For example, both the EU’s Medical Device Regulation (MDR) and NHS guidelines emphasize fairness, transparency, and security when using AI technologies.This reflects ongoing efforts towards creating regulations that can keep pace with rapid developments while promoting responsible and ethical use of these technologies in healthcare.
Legal liability and responsibility
The use of AI in healthcare raises concerns about legal liability and responsibility. Biased algorithms can create ethical dilemmas for both providers and institutions, potentially leading to negative consequences such as financial repercussions.
In order to prioritize patient autonomy and consent, it is important for legal frameworks to adapt accordingly. This includes ensuring that AI technologies protect patient privacy and uphold data security standards. As the integration of AI into healthcare becomes more prevalent, establishing clear guidelines on legal liability and responsibility is crucial not only for protecting patients, but also for safeguarding the interests of healthcare providers.
Future prospects and potential solutions
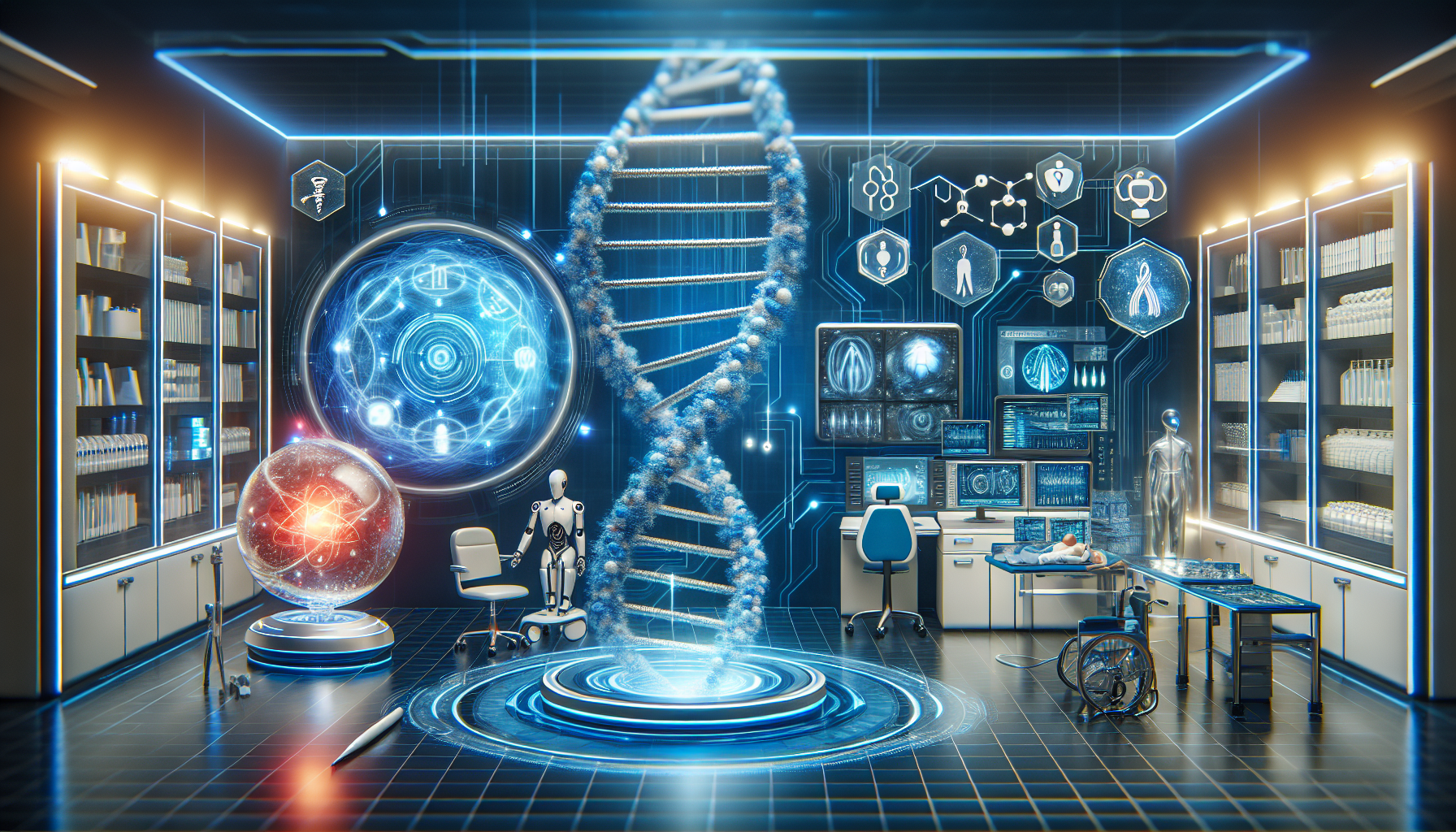
Looking to the future, the outlook for AI in healthcare is generally viewed as promising, indicating advancement and innovation in the field. Scientists today aim to develop a universally applicable AI with advanced and trustworthy algorithms to enhance healthcare delivery. However, the journey towards realizing the full potential of AI in healthcare is a balancing act. Managing the associated risks alongside the pursuit of innovative AI is crucial.
This balance can be achieved through a combination of emerging technologies, innovations, and collaborative efforts. By addressing the risks and challenges while advancing the field, we can harness the potential of AI to improve healthcare outcomes significantly, ushering in a new era of precision and personalized medicine.
Emerging technologies and innovations
The healthcare industry is witnessing the emergence of AI technologies and innovations that have potential to bring significant changes. These include personalized medicine, operational efficiency improvements, Emotion AI, and data management advancements.
AI has the ability to transform precision medicine by integrating omics data with patient electronic health records (EHRs) and input from wearable devices. This allows for individualized care regimens based on distinct patient characteristics.
In terms of improving hospital operations, AI contributes through enhanced scheduling algorithms and the use of robotic process automation, which minimizes errors in treatments and streamlines administrative tasks. Emotional AI is being applied in telemedicine applications to improve patient interaction and aid in diagnosing mental health conditions.
One area where noticeable progress has been made with regards to utilizing technology is managing healthcare data such as EHRs. With its capabilities in processing structured as well as unstructured types of information, artificial intelligence makes these records more accessible while also enhancing their analytical utility for better decision making processes within healthcare systems.
Collaborative efforts and best practices
Efforts to collaborate and utilize best practices are essential for addressing the risks and challenges posed by AI in healthcare. This involves establishing common standards, fostering cooperation, and promoting synergy among different stakeholders. The VALID AI collaboration is dedicated to these principles as it works towards ethical development of AI in healthcare. Through global knowledge sharing via co-published white papers, journal articles, and op-eds, this partnership aims to advance responsible use of AI technologies.
In order to enable personalized treatments within health systems through the integration of technology like AI, medical professionals work closely with health care IT solution providers. This highlights the importance of teamwork when implementing new technology within healthcare settings.
Together they can effectively tackle any potential risks or challenges that may arise from utilizing AI in a collaborative manner aimed at improving patient outcomes.
Summary
Overall, the use of AI in healthcare has great potential to bring about better patient care, increased operational efficiency and innovative advancements. There are challenges that must be navigated carefully, such as data privacy and security concerns, ethical dilemmas and implementation obstacles. The success of integrating AI into healthcare depends on effectively managing these risks while pursuing progress. By utilizing emerging technologies responsibly, promoting collaborative efforts and adhering to strong legal regulations, we can strive towards a future where AI seamlessly improves health outcomes while safeguarding patient privacy and trust.
Frequently Asked Questions
What are the risks of artificial intelligence in healthcare?
The use of artificial intelligence in healthcare introduces potential hazards, such as the risk of re-identifying individuals and susceptibility to data breaches and ransomware attacks. It is essential for both healthcare entities and their vendors to prioritize robust measures for ensuring data security.
What are the disadvantages of medical AI?
Medical AI has some drawbacks that should be considered, such as the possibility of data privacy issues and the potential danger of excessive dependence on technology for patient diagnosis.
How will healthcare be affected by AI?
The implementation of artificial intelligence (AI) in healthcare has the potential to assess medical information and forecast potential health hazards, resulting in proactive measures for prevention and ultimately leading to improved patient results while also reducing expenses. It is anticipated that this progress will have a substantial influence on the field of healthcare.
What is AI bias in healthcare, and what are its consequences?
AI bias in healthcare can lead to poorer health outcomes and perpetuate discrimination in patient care, particularly for underrepresented groups. It significantly impacts clinical decision-making.
What are the regulatory and legal aspects of AI in healthcare?
The use of AI in healthcare raises concerns regarding regulatory and legal frameworks, prompting the development of new laws and policies to effectively manage emerging challenges. Examples include initiatives like the FDA’s SaMD Action Plan which incorporates artificial intelligence/machine learning technology, guidelines from NHS for implementation in medical settings, and Medical Device Regulation by EU that addresses potential risks associated with advancing technologies.